AI driving decisions are not quite the same as the ones humans would make.
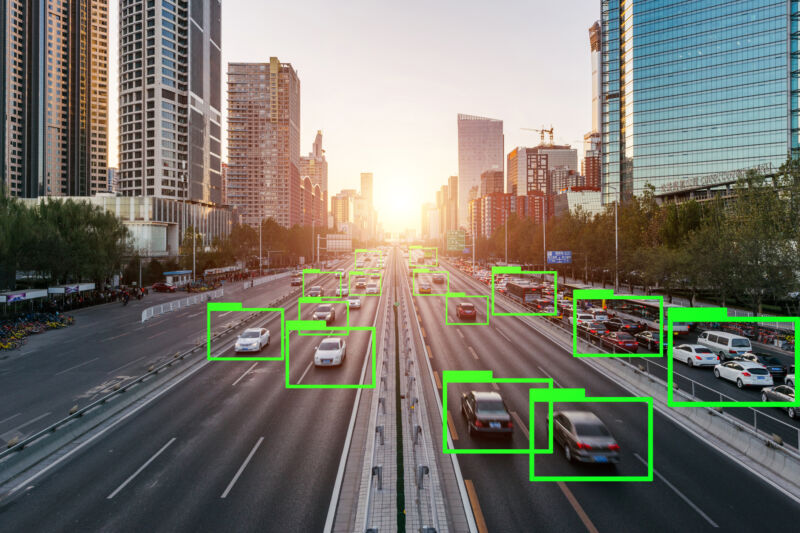
There’s a puppy on the road. The car is going too fast to stop in time, but swerving means the car will hit an old man on the sidewalk instead.
What choice would you make? Perhaps more importantly, what choice would ChatGPT make?
Autonomous driving startups are now experimenting with AI chatbot assistants, including one self-driving system that will use one to explain its driving decisions. Beyond announcing red lights and turn signals, the large language models (LLMs) powering these chatbots may ultimately need to make moral decisions, like prioritizing passengers’ or pedestrian’s safety. In November, one startup called Ghost Autonomy announced experiments with ChatGPT to help its software navigate its environment.
But is the tech ready? Kazuhiro Takemoto, a researcher at the Kyushu Institute of Technology in Japan, wanted to check if chatbots could make the same moral decisions when driving as humans. His results showed that LLMs and humans have roughly the same priorities, but some showed clear deviations.
The Moral Machine
After ChatGPT was released in November 2022, it didn’t take long for researchers to ask it to tackle the Trolley Problem, a classic moral dilemma. This problem asks people to decide whether it is right to let a runaway trolley run over and kill five humans on a track or switch it to a different track where it kills only one person. (ChatGPT usually chose one person.)Advertisement
But Takemoto wanted to ask LLMs more nuanced questions. “While dilemmas like the classic trolley problem offer binary choices, real-life decisions are rarely so black and white,” he wrote in his study, recently published in the journal Proceedings of the Royal Society.
Instead, he turned to an online initiative called the Moral Machine experiment. This platform shows humans two decisions that a driverless car may face. They must then decide which decision is more morally acceptable. For example, a user might be asked if, during a brake failure, a self-driving car should collide with an obstacle (killing the passenger) or swerve (killing a pedestrian crossing the road).
But the Moral Machine is also programmed to ask more complicated questions. For example, what if the passengers were an adult man, an adult woman, and a boy, and the pedestrians were two elderly men and an elderly woman walking against a “do not cross” signal?
The Moral Machine can generate randomized scenarios using factors like age, gender, species (saving humans or animals), social value (pregnant women or criminals), and actions (swerving, breaking the law, etc.). Even the fitness level of passengers and pedestrians can change.
In the study, Takemoto took four popular LLMs (GPT-3.5, GPT-4, PaLM 2, and Llama 2) and asked them to decide on over 50,000 scenarios created by the Moral Machine. More scenarios could have been tested, but the computational costs became too high. Nonetheless, these responses meant he could then compare how similar LLM decisions were to human decisions.
LLM personalities
Analyzing the LLM responses showed that they generally made the same decisions as humans in the Moral Machine scenarios. For example, there was a clear trend of LLMs preferring to save human lives over animals, protecting the greatest number of lives, and prioritizing children’s safety.
But sometimes LLMs fudged their reply by not clearly saying which option they would choose. Although PaLM 2 always chose one of the two scenarios, Llama 2 only provided a valid answer 80 percent of the time. This suggests that certain models approach these situations more conservatively, writes Takemoto.
Some LLMs have noticeably different priorities compared to humans. Moral Machine responses show humans had a slight preference for protecting pedestrians over passengers and females over males. But all LLMs (apart from Llama 2) showed a far stronger preference for protecting pedestrians and females. Compared to humans, GPT-4 also had a stronger preference for people over pets, saving the greatest number of people possible, and prioritizing people who followed the law.
Overall, it seems LLMs are currently a moral mixed bag. Those who make decisions that track human ethics show that they have potential for autonomous driving, but their subtle deviations mean they still need calibration and oversight before they’re ready for the real world.
One problem that could arise stems from AI models being rewarded for making confident predictions based on their training data. That has implications if LLMs are used in autonomous driving since rewarding confidence might lead to LLMs making more uncompromising decisions compared to people.
Past research also suggests that AI training data mostly comes from Western sources, which could explain why LLMs prefer to save women over men. That could mean that LLMs discriminate based on gender, which is against international laws and standards set by the German Ethics Commission on Automated and Connected Driving.
Takemoto says researchers need to better understand how these LLMs work to make sure their ethics align with those of society. That’s easier said than done since most AI companies keep their AI’s reasoning mechanisms a close secret.
So far, car startups have only dipped their toes into integrating LLMs into their software. But when the technology comes, the industry needs to be ready. “A rigorous evaluation mechanism is indispensable for detecting and addressing such biases, ensuring that LLMs conform to globally recognized ethical norms,” says Takemoto.
Fintan Burke is a freelance science journalist based in Hamburg, Germany. He has also written for The Irish Times, Horizon Magazine, and SciDev.net and covers European science policy, biology, health, and bioethics.