Gen-3 Alpha produces wild and whimsical results. Here’s what it cooked up for us.
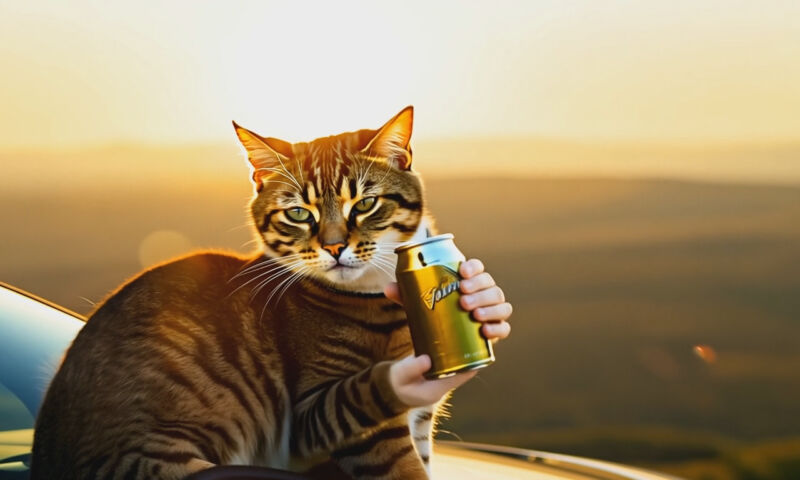
In June, Runway debuted a new text-to-video synthesis model called Gen-3 Alpha. It converts written descriptions called “prompts” into HD video clips without sound. We’ve since had a chance to use it and wanted to share our results. Our tests show that careful prompting isn’t as important as matching concepts likely found in the training data, and that achieving amusing results likely requires many generations and selective cherry-picking.
FURTHER READING
Runway’s latest AI video generator brings giant cotton candy monsters to life
An enduring theme of all generative AI models we’ve seen since 2022 is that they can be excellent at mixing concepts found in training data but are typically very poor at generalizing (applying learned “knowledge” to new situations the model has not explicitly been trained on). That means they can excel at stylistic and thematic novelty but struggle at fundamental structural novelty that goes beyond the training data.
What does all that mean? In the case of Runway Gen-3, lack of generalization means you might ask for a sailing ship in a swirling cup of coffee, and provided that Gen-3’s training data includes video examples of sailing ships and swirling coffee, that’s an “easy” novel combination for the model to make fairly convincingly. But if you ask for a cat drinking a can of beer (in a beer commercial), it will generally fail because there aren’t likely many videos of photorealistic cats drinking human beverages in the training data. Instead, the model will pull from what it has learned about videos of cats and videos of beer commercials and combine them. The result is a cat with human hands pounding back a brewsky.
(Update: Runway has not revealed where it got its training data, but after the publication of this article, 404 Media posted a report that seems to show that much of the video data came from an unauthorized scrape of YouTube videos.)
A few basic prompts
During the Gen-3 Alpha testing phase, we signed up for Runway’s Standard plan, which provides 625 credits for $15 a month, plus some bonus free trial credits. Each generation costs 10 credits per one second of video, and we created 10-second videos for 100 credits a piece. So the quantity of generations we could make were limited.
ARS VIDEO
How The Callisto Protocol’s Gameplay Was Perfected Months Before Release
We first tried a few standards from our image synthesis tests in the past, like cats drinking beer, barbarians with CRT TV sets, and queens of the universe. We also dipped into Ars Technica lore with the “moonshark,” our mascot. You’ll see all those results and more below.
We had so few credits that we couldn’t afford to rerun them and cherry-pick, so what you see for each prompt is exactly the single generation we received from Runway.
“A highly-intelligent person reading “Ars Technica” on their computer when the screen explodes”
“commercial for a new flaming cheeseburger from McDonald’s”
“The moonshark jumping out of a computer screen and attacking a person”
“A cat in a car drinking a can of beer, beer commercial”
“Will Smith eating spaghetti” triggered a filter, so we tried “a black man eating spaghetti.” (Watch until the end.)
“Robotic humanoid animals with vaudeville costumes roam the streets collecting protection money in tokens”
“A basketball player in a haunted passenger train car with a basketball court, and he is playing against a team of ghosts”
“A herd of one million cats running on a hillside, aerial view”
“video game footage of a dynamic 1990s third-person 3D platform game starring an anthropomorphic shark boy”
Some notable failures
The current state of Runway’s video synthesis tech already contains plenty of conceptual errors, as you’ve seen above. So that brings up a good question: What should we consider a generation failure when we are generally pleased that a cat suddenly sprouted a human hand while drinking a can of beer?
In this case, we feel that there were times when the AI model did not follow the prompt very closely—either thematically or suggested camera movements. And at a bare minimum, these generations failed to entertain us.
“Benj Edwards, a computer journalist, writing about AI on a typewriter that turns into a robot”
“fast motion zoom in and spin around a beautiful queen of the universe”
“A scared woman in a Victorian outfit running through a forest, dolly shot”
“a muscular barbarian with weapons beside a CRT television set, cinematic, 8K, studio lighting”
“aerial shot of a small American town getting deluged with liquid cheese after a massive cheese rainstorm where liquid cheese rained down and dripped all over the buildings”
Experimenting with more detailed prompts
Since building good prompts for Gen-3 can be tricky, someone created a GPT assistant (for ChatGPT) that can help convert simple prompts into more descriptive prompting language that includes more detailed camera instructions. Using that GPT, we created the following generations:
“Low angle static shot: A teddy bear sitting on a picnic blanket in a park, eating a slice of pizza. The teddy bear is brown and fluffy, with a red bowtie, and the pizza slice is gooey with cheese and pepperoni. The sun is setting, casting a golden glow over the scene”
“High angle static shot: A hacker in the 1980s wearing a gray hoodie, hunched over an Apple II computer in a dimly lit room with scattered cables and monitors. The screen displays lines of green code as the hacker types furiously, attempting to break into the Pentagon’s network. The room is bathed in the eerie glow of the computer screen and a small desk lamp”
“Wide-angle shot, starting with the Sasquatch at the center of the stage giving a TED talk about mushrooms, then slowly zooming in to capture its expressive face and gestures, before panning to the attentive audience.”
In the end, the fancy prompts didn’t really help. Runway Gen-3 Alpha is a psychedelic toy at the moment and can be entertaining if you can afford the credits. But it generally lacks the coherency to generate what might be called “useful video,” although your mileage may vary depending on the project. Even if the results were flawless, the ethics of using a video synthesis model trained on an unknown dataset might spawn some backlash.
FURTHER READING
AI-generated video of Will Smith eating spaghetti astounds with terrible beauty
What could improve Runway’s AI models? Among other things, more training data with better annotations. The AI model needs as many varied, well-labeled examples to learn from so it can do a better job of translating prompts into things a user would like to see. One of the reasons OpenAI’s GPT-4 turned heads in text synthesis is that the model finally reached a size where it was large enough to have absorbed enough information (in training data) to give the impression that it might be able to genuinely understand and model the world when, in reality, a key aspect of its success is that it “knows” far more than most humans and can impress us by combining those existing concepts in novel ways.
With enough training data and computation, the AI industry will likely reach what you might call “the illusion of understanding” with AI video synthesis eventually—but people who work in the TV and film production industries might not like it.