Will LLMs keep improving if we throw more compute at them? OpenAI dealmaker thinks so.
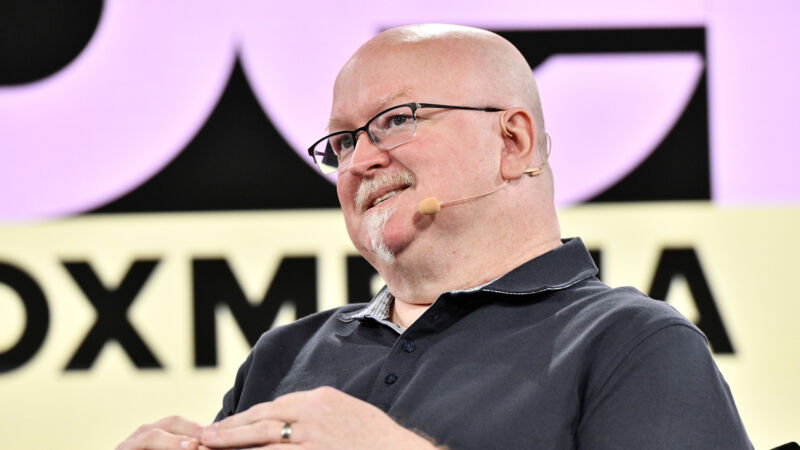
During an interview with Sequoia Capital’s Training Data podcast published last Tuesday, Microsoft CTO Kevin Scott doubled down on his belief that so-called large language model (LLM) “scaling laws” will continue to drive AI progress, despite some skepticism in the field that progress has leveled out. Scott played a key role in forging a $13 billion technology-sharing deal between Microsoft and OpenAI.
FURTHER READING
OpenAI training its next major AI model, forms new safety committee
“Despite what other people think, we’re not at diminishing marginal returns on scale-up,” Scott said. “And I try to help people understand there is an exponential here, and the unfortunate thing is you only get to sample it every couple of years because it just takes a while to build supercomputers and then train models on top of them.”
LLM scaling laws refer to patterns explored by OpenAI researchers in 2020 showing that the performance of language models tends to improve predictably as the models get larger (more parameters), are trained on more data, and have access to more computational power (compute). The laws suggest that simply scaling up model size and training data can lead to significant improvements in AI capabilities without necessarily requiring fundamental algorithmic breakthroughs.
Since then, other researchers have challenged the idea of persisting scaling laws over time, but the concept is still a cornerstone of OpenAI’s AI development philosophy.
You can see Scott’s comments in the video below beginning around 46:05:
Microsoft CTO Kevin Scott on how far scaling laws will extend
Scott’s optimism contrasts with a narrative among some critics in the AI community that progress in LLMs has plateaued around GPT-4 class models. The perception has been fueled by largely informal observations—and some benchmark results—about recent models like Google’s Gemini 1.5 Pro, Anthropic’s Claude Opus, and even OpenAI’s GPT-4o, which some argue haven’t shown the dramatic leaps in capability seen in earlier generations, and that LLM development may be approaching diminishing returns.
ARS VIDEO
What Happens to the Developers When AI Can Code? | Ars Frontiers
“We all know that GPT-3 was vastly better than GPT-2. And we all know that GPT-4 (released thirteen months ago) was vastly better than GPT-3,” wrote AI critic Gary Marcus in April. “But what has happened since?”
The perception of plateau
Scott’s stance suggests that tech giants like Microsoft still feel justified in investing heavily in larger AI models, betting on continued breakthroughs rather than hitting a capability plateau. Given Microsoft’s investment in OpenAI and strong marketing of its own Microsoft Copilot AI features, the company has a strong interest in maintaining the perception of continued progress, even if the tech stalls.
FURTHER READING
“The king is dead”—Claude 3 surpasses GPT-4 on Chatbot Arena for the first time
Frequent AI critic Ed Zitron recently wrote in a post on his blog that one defense of continued investment into generative AI is that “OpenAI has something we don’t know about. A big, sexy, secret technology that will eternally break the bones of every hater,” he wrote. “Yet, I have a counterpoint: no it doesn’t.”
Some perceptions of slowing progress in LLM capabilities and benchmarking may be due to the rapid onset of AI in the public eye when, in fact, LLMs have been developing for years prior. OpenAI continued to develop LLMs during a roughly three-year gap between the release of GPT-3 in 2020 and GPT-4 in 2023. Many people likely perceived a rapid jump in capability with GPT-4’s launch in 2023 because they had only become recently aware of GPT-3-class models with the launch of ChatGPT in late November 2022, which used GPT-3.5.
In the podcast interview, the Microsoft CTO pushed back against the idea that AI progress has stalled, but he acknowledged the challenge of infrequent data points in this field, as new models often take years to develop. Despite this, Scott expressed confidence that future iterations will show improvements, particularly in areas where current models struggle.
“The next sample is coming, and I can’t tell you when, and I can’t predict exactly how good it’s going to be, but it will almost certainly be better at the things that are brittle right now, where you’re like, oh my god, this is a little too expensive, or a little too fragile, for me to use,” Scott said in the interview. “All of that gets better. It’ll get cheaper, and things will become less fragile. And then more complicated things will become possible. That is the story of each generation of these models as we’ve scaled up.”